
Wales Products
Wales products are generated primarily from Sentinel-1 and 2 radar and optical data acquired between 2028 and 2023
Living Wales provides access to data products and also the algorithms used in their generation.
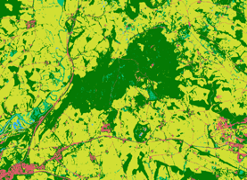
The Level 3 categories for Wales that are mapped are semi-natural terrestrial and aquatic vegetation and cultivated terrestrial vegetation, artificial and bare surfaces and water.
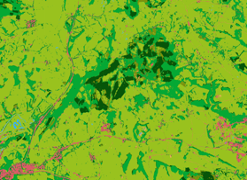
For Wales, forest leaf type and phenology is mapped using the algorithm of Punalekar et al. (2021). An ExtrTree machine learning algorithm is applied to Sentinel-2 composites, with this differentiating needleleaved deciduous (larch) and evergreen (other conifers) and broadleaved decisuous forests within areas mapped to the woody lifeform category. Evergreen broadleaved trees have a limited distribution in Wales with most existing as small isolated stands (e.g., of Holm Oak).
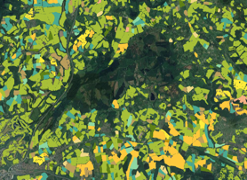
Living Wales differentiates crop types (currently for 2018) using time-series of Sentinel-1 radar data and tracking the changes in C-band backscatter with the structural development and management cycles of crops, with temporal differences allowing separation of eight crop types (winter and spring barley and wheat, rapeseed, maize, potatoes and beet).
The annual water hydroperiod for Wales is defined through temporal thresholding of time-series of Sentinel-1 data, with this allowing separation of permanent and temporary water (e.g., because of flooding). The moisture content of vegetation is also indicated, although the vegetation type (e.g., short grass) can influence the signal and hence the estimation of hydroperiod
Through the European Space Agency (ESA) Climate Change Initiative (CCI) Biomass global biomass project, above ground biomass of woody vegetation (in Mg/hectare) is estimated from time-series of C- and L-band radar, with 100 m spatial resolution maps subset for Wales being currently available for 2010, 2017, 2018 and 2020.
The habitat maps generated for Wales are generated by classifying vegetation typese within the area mapped as semi-natural vegetation through machine learning. These are then further divided using contextual information that cannot be mapped directly through Earth observations.